Satellite Image Time Series Semantic Change Detection: Novel Architecture and Analysis of Domain Shift
Elliot Vincent1,2
Jean Ponce3,4
Mathieu Aubry1
1Abstract
Satellite imagery plays a crucial role in monitoring changes happening on Earth's surface and aiding in climate analysis, ecosystem assessment, and disaster response. In this paper, we tackle semantic change detection with satellite image time series (SITS-SCD) which encompasses both change detection and semantic segmentation tasks. We propose a new architecture that improves over the state of the art, scales better with the number of parameters, and leverages long-term temporal information. However, for practical use cases, models need to adapt to spatial and temporal shifts, which remains a challenge. We investigate the impact of temporal and spatial shifts separately on global, multi-year SITS datasets using DynamicEarthNet and MUDS. We show that the spatial domain shift represents the most complex setting and that the impact of temporal shift on performance is more pronounced on change detection than on semantic segmentation, highlighting that it is a specific issue deserving further attention.
Proposed method
1. multiUTAE: a multi-temporal architecture for SITS-SCD
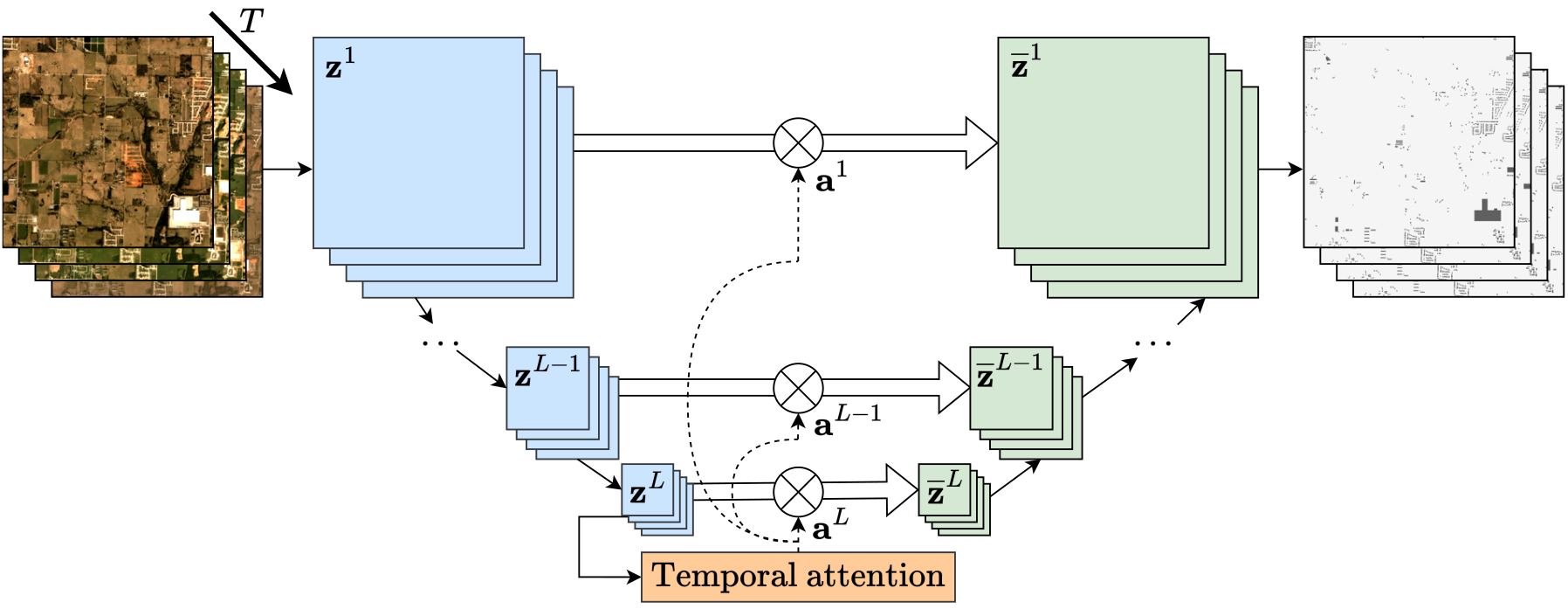
Overview. Given an input SITS, we compute feature maps at various scales. Our contribution is the temporal attention mechanism that allows to account for long-term temporal information. The decoder branch up-scales the feature maps for all time stamps in parallel, while propagating the attention maps at all levels.
2. Analysis of domain shift
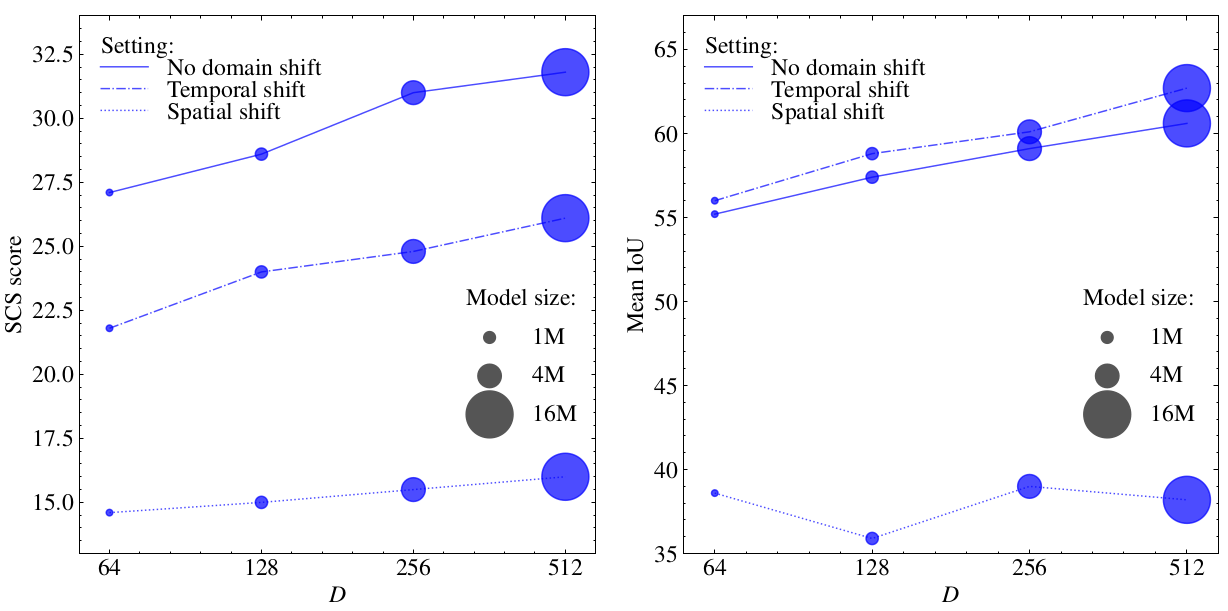
Domain shift analysis. We analyze the relation between the model size and performance for our method in different domain shift settings. Two effects are striking. First, there is no significant improvement in the spatial shift setting, highlighting the importance of spatial domain shift. Second, the semantic segmentation results are similar without domain shift and in the temporal shift setting, but change detection performance is clearly impacted by temporal domain shift.
BibTeX
If you find this work useful for your research, please cite:
@misc{ vincent2024satellite, title={Satellite Image Time Series Semantic Change Detection: Novel Architecture and Analysis of Domain Shift}, author={Elliot Vincent and Jean Ponce and Mathieu Aubry}, year={2024}, eprint={2407.07616}, archivePrefix={arXiv}, primaryClass={cs.CV} }
Acknowledgements
The work of MA was partly supported by the European Research Council (ERC project DISCOVER, number 101076028). JP was supported in part by the Louis Vuitton/ENS chair on artificial intelligence and the French government under management of Agence Nationale de la Recherche as part of the Investissements d’avenir program, reference ANR19-P3IA0001 (PRAIRIE 3IA Institute). This work was granted access to the HPC resources of IDRIS under the allocation 2021-AD011013067 made by GENCI. We thank Antoine Guédon, Loïc Landrieu and Ioannis Siglidis for valuable feedbacks, and Zeynep Sonat Baltaci and Syrine Kalleli for their careful proofreading.